- 2024-11-14
- News
Big Models Shift Focus from Scaling Law to Diversified Exploration
Since the launch of OpenAI's new AI inference model GPT-01 in September, domestic enterprises have begun a flurry of updates to align their processes with inference models.
On the evening of November 25th, the Shanghai Artificial Intelligence Lab opened its Shusheng·Puyu model to social users, launching the powerful inference model InternThinker on the interface. It is reported that the InternThinker model possesses the ability for extended reasoning and can engage in reflection and correction during the inference process, achieving superior results in complex reasoning tasks such as mathematics, coding, and logical puzzles.
This month has seen the release of several inference models. On November 16th, the Dark Side of the Moon's Kimi announced the launch of its next-generation mathematical reasoning model, k0-math, which claims to rival the mathematical capabilities of OpenAI's O1 series. On November 20th, DeepSeek released the inference model DeepSeek-R1-Lite, asserting that it performs comparably to O1-preview in mathematical, coding, and complex logical reasoning tasks, thanks to reinforcement learning training.
Advertisement
The release of inference models has become a significant trend in the current AI industry. Chen Kai, a young scientist at the Shanghai Artificial Intelligence Lab, stated in an interview with Yicai that “leading organizations in large models are all planning to develop and release inference models because reasoning ability is an important indicator of the intelligence level of large models and is an essential capability for tackling complex application scenarios.”
Robust reasoning capabilities form an essential foundation for advancing towards general artificial intelligence. From an application standpoint, Chen believes that further enhancement of model inference abilities will yield a plethora of intelligent application scenarios, enabling better collaboration with humans in thinking and solving challenging tasks, thus promoting the productivity applications of large models.
In practical applications, Chen provides an example, stating that a typical large model can help summarize key information after reading a financial report, whereas a model equipped with strong inference capabilities could, in the future, assist analysts in analyzing data from financial reports, offering sound research and predictions.
Regarding the improvement of model inference ability, Chen mentioned that the current main challenge lies in obtaining high-density supervised data, such as difficult questions and more detailed reasoning chains, which constitute a small fraction of natural text. It is essential to research effective construction methods for such data. Additionally, the path to enhancing reasoning ability relies on effective reinforcement learning; thus, improving the model's search efficiency and training a generalizable and reliable reward model for obtaining feedback also presents challenges.
The O1 model released by OpenAI in September showcased powerful reasoning capabilities. In their research aimed at improving model inference abilities, the lab is reportedly taking a relatively independent route by designing a meta-action thinking paradigm to guide the model's search space, synthesizing data through a fusion of general and specialized approaches, and creating a large-scale sandbox environment to gather feedback, thereby enhancing the model's performance.
Specifically, when humans learn to solve complex reasoning tasks, it is not mere point-based knowledge acquisition from a vast array of samples; rather, it is about learning thinking patterns—through recalling relevant knowledge, understanding and remembering the correct problem-solving process, and reflecting on and correcting erroneous approaches. This involves being aware of and regulating one's cognitive processes. This capability is often referred to as metacognitive ability.
Inspired by metacognitive theories, the lab's research team designed a series of meta-actions to guide the model through problem-solving processes, such as understanding the problem, recalling knowledge, planning, executing, and summarizing. When faced with complex tasks, the model will explicitly and dynamically select meta-actions while elaborating on the specific thought processes for related actions. This design reinforces the model's utilization of key combinations of meta-actions through part of the training tasks, thus enhancing the model's learning efficiency.
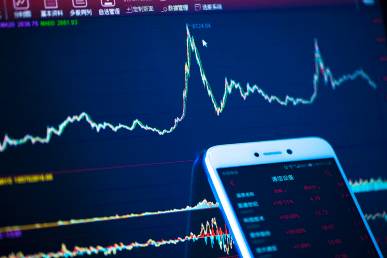
With the continuous development of large models, Chen believes the current research direction in the industry is shifting from simply scaling model parameters and data according to the Scaling Law to broader explorations. He predicts that in the future, a portion of resources will shift from pre-training to post-training, including utilizing more reasoning computational power to achieve better model performance and the large-scale application of reinforcement learning.
Earlier, when discussing whether the Scaling Law remains effective upon releasing its inference model, Kimi's founder and CEO Yang Zhilin also mentioned a paradigm shift in Scaling Law. He posits that the previous path for large models followed the "next token prediction" approach. However, predicting the next word has limitations as it is based on a static dataset that cannot explore more challenging tasks. The upcoming goal for large models is to empower AI with reasoning capabilities through reinforcement learning.
“The ability to continue scaling still exists, just through a different process,” Yang Zhilin believes that there is still room for half a generation to a generation of models in pre-training, which may be unlocked next year. However, he judges that the primary focus moving forward will be reinforcement learning.
- 109 Comments
- 113
- 119